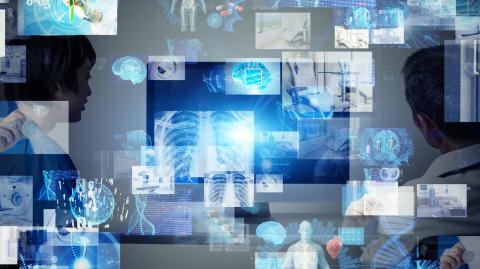
AI for Covid-19: personalised and predictive care for patients
A group of doctors and researchers from Gustave Roussy, Université Paris-Saclay, the Bicêtre Hospital – AP-HP, the French Institute for Research in Computer Science and Automation (INRIA) and the start-up Owkin have recently developed a new AI-based severity score that can predict outcomes for Covid-19 patients as soon as they are diagnosed. Its open source code has been published in the review Nature Communications.
The progression of Covid-19 in patients varies greatly. Being able to predict a patient’s risk of deterioration at diagnosis, i.e. whether they will require respiratory assistance or need to be transferred to intensive care, is imperative. As a result, doctors and researchers from Gustave Roussy, Université Paris-Saclay, the Bicêtre Hospital – AP-HP, INRIA and the start-up Owkin have come together to tackle this urgent issue.
The new AI tool produces a severity score that combines a range of variables to predict the patient's progression. Calculating the score takes just two to three minutes and can be given to doctors at the same time as the scan report for each patient assessed. The tool has recently been clinically implemented within Gustave Roussy's Radiology Department.
Ranging from 1 (for the lowest risk) to 5 (for the highest risk), the score alerts doctors and allows them to adapt how the patient is monitored to anticipate deterioration. Consequently, the tool helps to provide a more personalised therapeutic management of Covid-19 patients.
The severity score was developed as part of the ScanCovIA study led by Prof Nathalie Lassau, radiologist at Gustave Roussy, and conducted in close collaboration with teams from Gustave Roussy, Université Paris-Saclay, the Bicêtre Hospital – AP-HP, INRIA and Owkin.
The study is based on the cross-analysis of various clinical, biological and radiological variables using artificial intelligence. The study also makes use of a key tool - chest CT scans, which assess the extent and nature of lesions in the thorax and diagnose lung damage.
Accurately calculating the severity score
The deep learning AI model was trained and then validated on over 1,000 patients, simultaneously analysing and combining heterogeneous data from CT scans, clinical and biological data, as well as patients’ medical history and comorbidities. Of the 65 variables analysed, five proved to be particularly important in determining the prognosis: oxygen saturation, platelet counts (medullary function index), urea (renal impairment), age and gender.
By combining these five variables and CT scans, the tool is able to accurately calculate a severity score that can categorise patients according to their possible outcome, the likelihood of them being transferred to intensive care, and whether they will require respiratory assistance, etc. The tool helps doctors answer essential questions related to urgent care and predict patients’ needs and therapeutic options.
The Radiology Department at Gustave Roussy has been using the tool for the past month and it has already proven useful in the clinical management of Covid patients. The clinical implementation of this AI tool in just six months is an excellent example of the advancement of research for patients during the COVID-19 pandemic.
In the article published in Nature Communications, a comparative study ranked ScanCovIA's AI model as the best performing AI tool out of 11 studies published to date. Its open source code can be used by imaging departments in France and around the world.
The study has benefited from the support of donors, including Malakoff Humanis.
Integrating deep learning CT-scan model, biological and clinical variables to predict severity of COVID-19 patients
Nature Communications, published online 27 January 2021 - DOI: 10.1038/s41467-020-20657-4
Nathalie Lassau1,2, Samy Ammari1,2, Emilie Chouzenoux3, Hugo Gortais4, Paul Herent5, Matthieu Devilder4, Samer Soliman4, Olivier Meyrignac4, Marie-Pauline Talabard4, Jean-Philippe Lamarque1,2, Remy Dubois5, Nicolas Loiseau5, Paul Trichelair5, Etienne Bendjebbar5, Gabriel Garcia1, Corinne Balleyguier1,2, Mansouria Merad6, Annabelle Stoclin7, Simon Jegou5, Franck Griscelli8, Nicolas Tetelboum1, Yingping Li2,3, Sagar Verma3, Matthieu Terris3, Tasnim Dardouri3, Kavya Gupta3, Ana Neacsu3, Frank Chemouni7, Meriem Sefta5, Paul Jehanno5, Imad Bousaid9, Yannick Boursin9, Emmanuel Planchet9, Mikael Azoulay9, Jocelyn Dachary5, Fabien Brulport5, Adrian Gonzalez5, Olivier Dehaene5, Jean-Baptiste Schiratti5, Kathryn Schutte5, Jean-Christophe Pesquet3, Hugues Talbot3, Elodie Pronier5, Gilles Wainrib5, Thomas Clozel5, Fabrice Barlesi6, Marie-France Bellin2,4, Michael G. B. Blum5
1.Imaging Department Gustave Roussy. Université Paris Saclay, Villejuif, F-94805
2.Biomaps. UMR1281 INSERM, CEA, CNRS, Université Paris-Saclay. Villejuif, F-94805
3.Centre de Vision Numérique, Université Paris-Saclay, CentraleSupélec, Inria, 91190 Gif-sur-Yvette, France
4.Radiology Department, Hôpital Bicêtre – AP-HP, Université Paris Saclay, Le Kremlin-Bicêtre, France
5.Owkin Lab, Owkin, Inc. New York, NY USA
6.Département d'Oncologie Médicale, Gustave Roussy, Université Paris-Saclay, Villejuif, F-94805, France
7.Département Interdisciplinaire d’Organisation des Parcours Patients, Service de Medecine intensive réanimation, Gustave Roussy, Université Paris-Saclay, Villejuif, F-94805, France
8.Département de Biologie, Gustave Roussy, Université Paris-Saclay, Villejuif, F-94805, France
9.Direction de la Transformation Numérique et des Systèmes d'Information, Gustave Roussy, 94800 Villejuif, France.