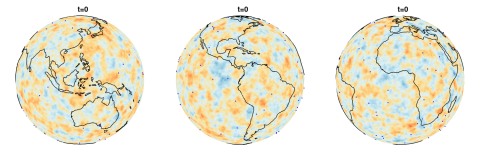
Lucia Clarotto: using geostatistics to predict environmental phenomena
Lucia Clarotto is a lecturer at the Applied Mathematics and Informatics Laboratory (MIA - Univ. Paris-Saclay, AgroParisTech, INRAE). With her expertise in geostatistics, she analyses and models complex spatio-temporal data, contributing to a better understanding and prediction of environmental phenomena.
Lucia Clarotto, originally from Italy, developed a passion for mathematics due to the influence of enthusiastic teachers. This interest, combined with her desire to apply mathematics to practical problems, led her to pursue graduate studies in this field. She earned a bachelor's degree in mathematical engineering from Politecnico di Milano in 2016 and continued with a double degree at École Centrale de Lyon in 2018. Her career then took her to Michelin's research and development department in South Carolina for an internship focusing on car accident prevention. In 2020, Lucia Clarotto obtained a master's degree in mathematical engineering with a specialisation in applied statistics, again in Milan, before completing a research internship in spatial and compositional statistics at the Biostatistics and SPatial Processes (BioSP) unit at INRAE in Avignon. In 2023, she presented her thesis in geostatistics and applied probabilities at the École Nationale Supérieure des Mines de Paris. Starting in September of the same year, she joined AgroParisTech as a lecturer and simultaneously conducts research at the MIA Paris-Saclay laboratory.
Spatio-temporal modelling for environmental predictions
Lucia Clarotto's PhD, entitled Spatio-Temporal Prediction by Stochastic Partial Differential Equations, was inspired by phenomena such as wind and the concentration of fine particles. It addressed the challenge of modelling partially observed data using probabilistic models based on spatial and temporal dependency structures. "I have developed models and methods that revisit the assumptions of traditional approaches in spatio-temporal statistics, such as separability and stationarity." Her method was based on stochastic partial differential equations (SPDEs) and in particular advection-diffusion SPDEs. This is a concept that describes the transport and dispersion of a substance or property within a fluid, incorporating a physical dimension to enhance the accuracy of predictions. Applied to the analysis of large environmental spatio-temporal datasets - combining statistics, physics and numerical analysis - this model proves to be more effective in terms of prediction than standard models. "It has the potential to facilitate the analysis and forecasting of environmental evolution and climatic variables, key elements of the ecological transition." Lucia Clarotto used this method to predict short-term solar radiation, in association with the Observation, Impacts, Energy (OIE) Centre at the École Nationale Supérieure des Mines de Paris. "Reliable and rapid prediction of such variables is not only interesting for environmental researchers, but also for the efficient management of the electricity grid."
Geostatistics and deep learning
Having recently joined the MIA Paris-Saclay laboratory after her PhD, Lucia Clarotto is focusing on applying deep learning to the analysis of complex data. She is developing innovative models by highlighting the improvement in the analysis and prediction of spatio-temporal phenomena. "My work involves a close interaction between multiple interdependent variables, typical of environmental data." She is also an active participant in a working group dedicated to advances in this field. Furthermore, she draws on her work on SPDE approaches and recent innovations in statistics and deep learning, such as Deep Gaussian Markov Random Field for probabilistic spatial models and neural ordinary differential equations for continuous dynamic modelling, to adapt them to spatio-temporal data. She is committed to exploring the practical applications of her methodological research, with the aim of integrating them into the life sciences.
International achievements and collaborations
Along with a team of four researchers from the École Nationale Supérieure des Mines de Paris and INRAE Avignon, Lucia Clarotto won the Spatial Statistics Competition for Large Datasets in both 2021 and 2022. This success earned her a five-week research stay at KAUST University in Saudi Arabia. "This experience opened my eyes to the value of diversity in backgrounds, genders and nationalities in research and today encourages me to promote international scientific collaboration." In addition, Lucia Clarotto actively disseminates her work, both within the scientific community and to a broader audience. An excellent communicator, she has received positive feedback at international conferences and seminars, as well as at events related to the entrepreneurial sector. For example, she plans to give a presentation on spatio-temporal statistics and the SPDE approach at the Statistical Workshop of the French Statistical Society in November 2024.
Teaching and encouraging young women in science
Lucia Clarotto teaches statistics at AgroParisTech, Université Paris-Saclay, Ecotrop (an AgroParisTech and University of Guyana programme dedicated to biodiversity, ecology and evolution), and at the École des Mines de Paris. "I aim to make students aware of the usefulness of statistical modelling, even for those who are not primarily focused on mathematics." Aware of the declining interest in mathematics among young women, especially in the context of its growing association with the digital sciences, she sees teaching at a predominantly female engineering school as an opportunity to change their perception. "I am convinced that examples of female success in science can encourage young women to get involved in these disciplines." She is therefore committed to contributing to a change in perspective in traditionally male-dominated fields, where the scientific merits of each individuals can be recognised regardless of gender.
Future collaborations
Looking ahead, Lucia Clarotto plans to work closely with the Geolearning Chair at the École Nationale Supérieure des Mines de Paris and INRAE. This Chair aims to develop modern, efficient tools for data analysis in support of the ecological transition. "My commitment to this project stems directly from my thesis, which I wrote in preparation for the establishment of this Chair. I have already played an active part in various events associated with this initiative." In addition, within the Risks, Extremes and Spatio-Temporal Statistics (RESSTE) network, dedicated to scientific exchange on models, methods and algorithms for spatio-temporal data, the lecturer aspires to contribute to the development of new research and the establishment of innovative collaborations.