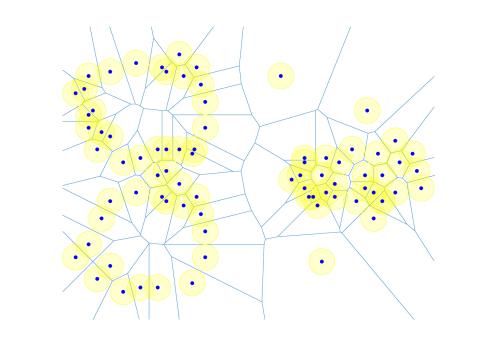
Nina Otter: a distinguished career in topological data analysis
Nina Otter is an Inria researcher and member of the Mathematics Laboratory of Orsay (LMO - Univ. Paris-Saclay, CNRS). She is a specialist in topological data analysis, and builds bridges between previously distinct mathematical fields, thereby showing her creative, interdisciplinary approach.
Nina Otter began her studies at the Swiss Federal Institute of Technology in Zurich (ETH) in 2008, initially in physics, before realising the importance of mathematics in understanding fields such as electrodynamics and classical mechanics. She switched to mathematics and wrote a dissertation on tropical elliptic curves. She completed a Master's degree in pure mathematics in the desire to combine this expertise with a commitment to societal issues. Influenced by John Baez's work on the application of mathematics in biology, she joined him at the University of California, where she wrote her Master's thesis in 2014. "As one of the few women in my field, I had to overcome my doubts about my ability to pursue a career in mathematical research, but John Baez's encouragement was instrumental in boosting my confidence." Their collaboration resulted in a publication on the application of categorical methods in phylogenetics.
A thesis in topological data analysis
In 2018, Nina Otter presented a thesis at Oxford University entitled Data Homology, which focuses on the development of topological tools for efficiently analysing complex data. Her work revolved around persistent homology, a method for identifying and quantifying various structures such as clusters or gaps. In 2017, this project resulted in a publication evaluating and comparing various software implementations of the method. "In the second part of my thesis, I adapted these methods to multiparametric data, a major challenge for real-world applications as it involved dealing with the complexity and interactions inherent in real datasets." This difficulty is one of the major challenges of topological data analysis, given the absence of invariants capable of generalising persistent homology for multiparametric data sets. To meet this challenge, Nina Otter employed commutative algebra techniques to design new invariants, thereby enriching the analysis of complex data structures. She also explored the connections between persistent homology and magnitude theory - introduced in 2010 by mathematician Tom Leinster to measure the size of a mathematical object - to create a new homological theory.
Merging topological data analysis and deep learning
After her PhD, Nina Otter spent six months at the Max Planck Institute in Leipzig, before joining the University of California at Los Angeles (UCLA) for post-doctorate studies. She explored the potential interactions between deep learning and topological data analysis, a subject that was captivating the scientific community. His first project, carried out with a collaborator, aimed to determine whether neural network architecture is capable of learning to generate topological summaries obtained by persistent homology, despite their non-linear complexity. "To my surprise, we managed to train some networks to learn these summaries in specific situations." This collaboration then extended to further projects with scientists from UCLA, the Max Planck Institute and the University of Cambridge, with a focus on adapting neural architectures to analyse combinatorial structures that are more complex than simple binary relations. These initiatives opened up new perspectives in geometric deep learning, and have a major influence on the modelling of natural and social phenomena, such as the COVID-19 pandemic.
Delving deeper into persistent homology
In July 2021, Nina Otter joined Queen Mary University of London as Assistant Professor. She started work on the innovative project of elucidating the effectiveness of persistent homology. "Despite its dominant presence in topological data analysis, this approach leads to a certain amount of wariness in the scientific community due to a potential excessive hype." In collaboration with colleagues at UCLA, she explored the ability of this method to solve complex analytical problems, even in tasks previously considered inaccessible. "We were delighted to discover that it manages to solve problems considered beyond its reach." New theoretical conclusions were reached, including the ability to distinguish between concave and convex shapes. "Unlike deep learning methods that are often seen as black boxes, persistent homology provides a clear understanding of the characteristics of the data that influence results." Nina Otter planned to further explore why and how this method works effectively for various problems, drawing on research into interpretability in deep learning.
Innovation and inclusion in scientific research
In March 2021, Nina Otter founded DeMoS, an association that aims to transform scientific research methods in order to meet modern challenges. This initiative promotes interdisciplinary approaches, combining science and the arts, and focuses on the inclusion of researchers from diverse backgrounds, including those generally excluded from scientific discourse due to linguistic, geographical or social discrimination.
Innovative climate research
Motivated by a less favourable post-Brexit academic context for scientists, the researcher joined Inria Saclay's DataShape team at the LMO in October 2023. "This is an ideal opportunity to pursue my research, as the team is world-renowned in the field of topological data analysis." Here, Nina Otter is developing advanced methods for improving climate modelling, to provide a better understanding and prediction of climatic phenomena. This is a new perspective on modelling complex atmospheric phenomena, a subject that has been difficult to tackle until now due to the lack of universal definitions of weather patterns. From this perspective, Nina Otter also explores the quasi-periodicity of the El Niño phenomenon. "In addition to the high dimensionality and noise inherent in climate data, the time-changing nature of this data leads to unique challenges for topological analysis."
Generalising methods for analysing complex networks
Nina Otter plans to continue the research she began in her post-doctorate studies by applying topological data analysis to complex social networks. The aim is to integrate the binary and higher-order relationships needed to characterise positions and roles within these systems. It deals with simplifying the understanding of these structures by grouping similar elements together, in order to facilitate the revelation of underlying patterns. "Networks are versatile tools for modelling a variety of phenomena, including molecular interactions, traffic flow and even climate time series. This provides promising prospects for a better understanding and analysis of complex systems."